👶 Voices from the Delivery Room: A Hindi Speech Dataset Built for Life-Saving ASR
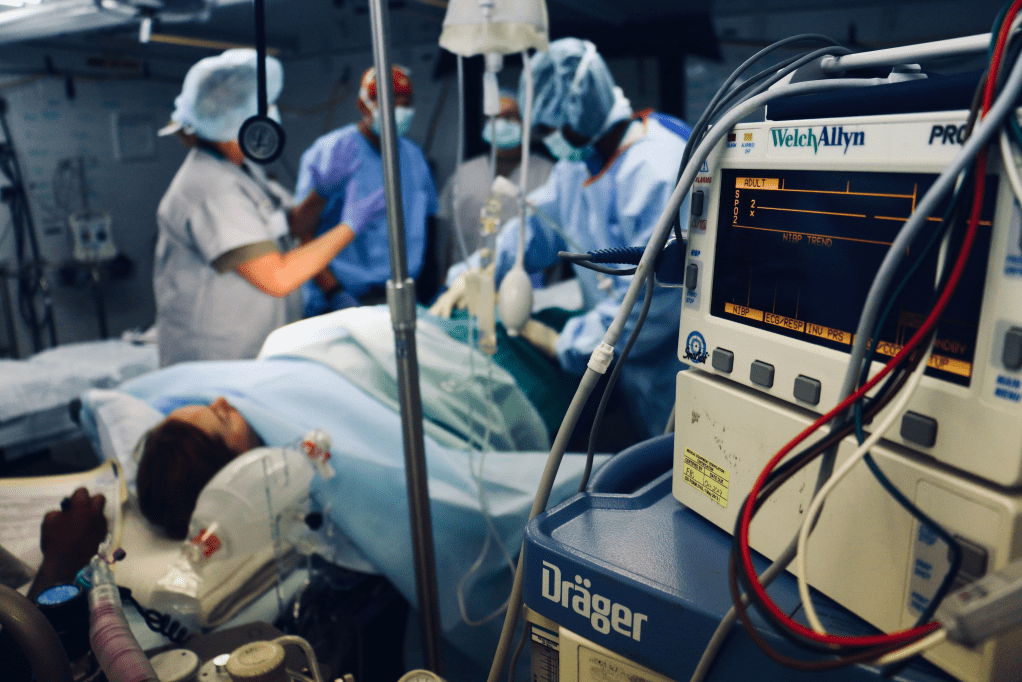
💡 What’s This About?
Automatic Speech Recognition (ASR) is everywhere — from Siri to voice typing. But what if we could use ASR to save lives?
This research introduces a custom Hindi speech dataset designed to help nurses in rural Bihar transcribe medical data during childbirth — where hands are busy, time is limited, and typing is just not an option.
🏥 The Challenge: Speech Recognition in the Chaos of a Delivery Ward
Imagine trying to use a voice assistant in a noisy hospital room while helping deliver a baby.
Here’s what makes the task hard:
- Noisy environments with patients, cross-talk, and urgent tasks.
- Accents from a specific region (Bihar) not covered in standard datasets.
- Code-switching between Hindi and English.
- Medical terms like “dilatation,” “FHR,” “effacement,” etc., not common in everyday speech.
- Inconsistent pronunciation and casual speech patterns.
Off-the-shelf voice models struggle in this setting. That’s why the team built their own dataset: Parturition Hindi Speech (PHS).
📦 What’s Inside the Dataset?
- 🗣️ 2000 audio samples, around 10 seconds each (~5.6 hours total).
- 👩⚕️ Spoken by real nurses in hospitals, with natural speech quirks.
- 📃 Comes with Devanagari script and Romanized transcripts.
- 🧪 Focused on 8 key medical parameters like blood pressure (BP), pulse, temperature, etc.
- 🎙️ Recorded with close-talking mics in real delivery and triaging rooms.
This is a limited-vocabulary, domain-specific dataset — small, but powerful.
🔧 How Was It Built?
- Corpus Generation
Sentences were generated by combining common delivery parameters like “BP 113/61” or “FHR 120.” Values were statistically modeled to simulate real medical readings. - Real Hospital Recordings
Nurses read these sentences while not performing delivery, but still in the actual acoustic environment of maternity wards. - Ethics and Accuracy
- Data collection followed ethical protocols and informed consent.
- Transcriptions were validated by humans and ASR feedback.
- Final dataset is clean, accurate, and anonymized.
- Data collection followed ethical protocols and informed consent.
🎯 Why This Is Important
In delivery rooms, timing is everything, and manually jotting down observations isn’t always feasible.
This dataset:
- Makes hands-free recordkeeping possible.
- Is built for Hindi + English spoken with a Bihari accent.
- Uses real-world hospital noise, making it incredibly robust.
- Can help build ASR systems for any critical, low-resource domain — especially in rural or underrepresented areas.
🤖 The Tech: Training and Results
They tested two types of ASR models:
🧪 1. Traditional Model: GMM-HMM
- Uses statistical modeling of phonemes.
- WER (Word Error Rate):
- Devanagari: 18.84%
- Romanized: 21.77%
- Devanagari: 18.84%
⚙️ 2. End-to-End Deep Learning Model
- Trained with pretrained NeMo model (QuartzNet).
- Adapted to this dataset using fine-tuning.
- WER:
- Without language model: 12.01%
- With language model: 2.7% 🎯
- Without language model: 12.01%
That’s a dramatic improvement with domain adaptation.
🧠 TL;DR: A Voice Model Made for Real-Life Birthrooms
This paper doesn’t just introduce a new dataset — it offers a blueprint for building voice recognition systems that actually work in real, noisy, high-stakes environments, like hospitals in rural India.
It’s not just about tech — it’s about making AI tools work where they’re needed most.